Data Lifecycle Management (DLM) | What Is It?
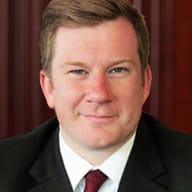
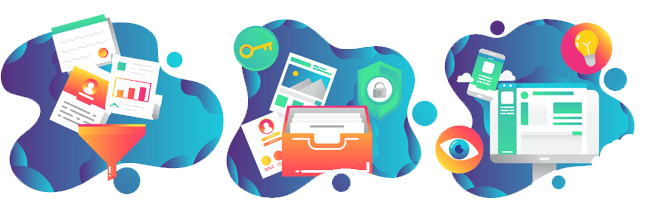
Data Lifecycle Management Overview
Data lifecycle management (DLM) refers to the best practices management of data in an organization from creation to archiving with the goal of achieving data integrity. While the type of data may vary greatly between industries like pharmaceuticals to construction to food production, the central tenets of data lifecycle management remain. Without the proper policies and data governance controls in place to manage and use data, data integrity can not be guaranteed.
Data lifecycle management is a broad area and even includes things like paper and recordable media. But we’ll focus here on data as it exists in a digital form.
Data Lifecycle Management: Elements of a Data Lifecycle
Data Lifecycle Plan
Construction of an overarching methodology and implementation of data needed, data collection, standards, management, analysis, accessibility, archiving, and destruction.
Data Collection
Rules and forms for gathering consistent, standardized, relevant data in a repeatable manner.
Data Processing and Maintenance
Data quality assurance requires inspection, review, and qualified sign-off on new data. Also scheduled review of existing data.
Data Publishing
Where, when, how, and to whom data is published internally and externally. Also how the data is described and stored along with metadata.
Data Usage
Data is organized clearly and can be searched/located easily by stakeholders.
Data Analysis
Data is cleaned, inspected, and transformed to find potentially useful or clarifying conclusions.
Data Archiving
Retiring data that are no longer useful or needed in long-term storage, separated from actively-used data.
Data Purging
Based on the rules governing your industry, administrators may consider data deletion to reduce security risks, save money on storage, or reduce potential confusion.
Workflow Automation and Data Lifecycle Management
Using a workflow automation platform to automate the acquisition, routing, review, approval, and archiving of data can have a drastic, positive impact on data lifecycle management.
Data Capture Forms
Smart forms can provide a consistent way of acquiring data across the organization. By capturing information, metadata, documents, and files accurately on the front end of the data lifecycle, stakeholders can be confident that their capture efforts provide accurate information. By using required fields, pre-fills, database integration, form logic, and a variety of other smart form capabilities, users providing data have a user-friendly but rule-based way to provide all the information that will be needed.
Data Routing
Manual routing of information is slow and subject to mistakes. To optimize the flow of data through the organization, an automated routing system is critical. Data can be routed to people and systems based on pre-set rules, staff hierarchies, and information acquired via capture forms. Alerts, reminders, and escalations ensure that each step in the data lifecycle is optimized and acted upon.
Review and Approval
Workflow administrators can define, create and adjust compliant approval workflows or approval steps as needs and regulations change. Every action taken in the workflow is tracked and available to audit including name, date, time, action taken, documents, forms, etc. Administrators can force a second login for any user, ensuring their identity.
Data and Document Archiving
Any documents and files can easily be pushed into the document management system or file system of record at any point in the automated workflow. That gives administrators the flexibility to use whatever storage system they’re most comfortable with and have come to rely on.
Data Lifecycle Management (DLM) FAQ
-
What is data lifecycle management (DLM)?
DLM is the process of managing data from creation to archiving, ensuring data integrity, quality, and security throughout its lifecycle.
-
Why is DLM important for organizations?
Proper DLM ensures consistent data quality, compliance with regulations, efficient data usage, and reduces security risks and storage costs.
-
What are the key stages in the data lifecycle?
Key stages include data collection, processing, publishing, usage, analysis, archiving, and purging, each with specific practices for data management.
-
How does workflow automation enhance DLM?
Workflow automation optimizes data capture, routing, review, approval, and archiving processes, ensuring consistent and efficient data management.
-
What are the benefits of automating data lifecycle management?
Benefits include improved data quality, reduced manual errors, compliance with data governance policies, and streamlined data management processes.
Interested in Automating Your Data Lifecycle Management Workflow?
We have a variety of resources to help you on your journey to an automated data life cycle workflow.