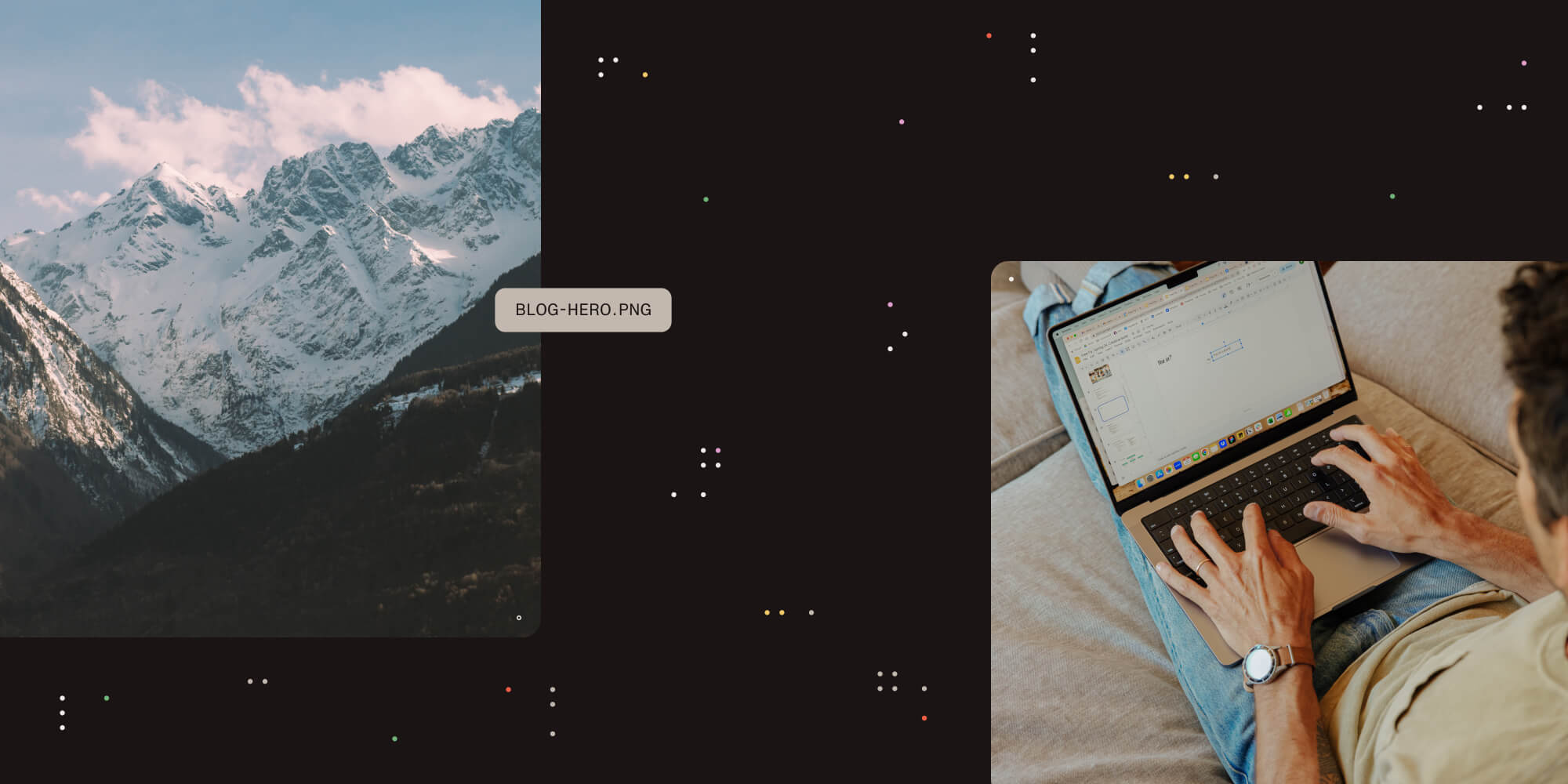
Aquaforest Searchlight will help you convert all your ‘hidden’ documents to searchable ones, thus making them discoverable. This is the first step in improving document discoverability in SharePoint. In order to make it easier to find the most relevant information, it is important to tag documents with the correct metadata. While SharePoint offers different ways of adding metadata to contents through the Managed Metadata Service and the SharePoint Term Store, they all require some form of continuous involvement of end-users and/or IT personnel. Consequently, they can be very time consuming & labour-intensive and thus prone to human error. Likewise, they do not scale well since maintaining a large number of metadata (term sets) can be very problematic. All these impact the accuracy and quality of the metadata. Having inaccurate metadata is as bad (if not worse) than having no metadata at all. According to a study by The International Data Corporation (IDC), the average knowledge worker wastes 2.5 hours per day, or about 30% of the workday searching for information. In this increasingly data-driven world, where accurate metadata can not only enrich contents but also enhance search, navigation and governance, automated semantic tagging is the most efficient solution.
TermSet
TermSet is a cloud based solution hosted in Microsoft Azure that uses artificial intelligence, machine learning & Natural Language Processing to enrich documents and items in SharePoint by extracting and applying consistent & accurate metadata to them without the need for IT to prepare taxonomies or for end users to manually apply metadata. There are 3 phases that TermSet uses to enrich contents in SharePoint:
All the above can be easily managed through the TermSet user interface:
To see how TermSet can enrich documents in SharePoint, let’s look at a very basic example. Below is a sample library with scanned pharmaceutical documents (TIFFs and image-only PDFs). Currently, the documents in the library do not have any metadata.
If we audit the library with Aquaforest Searchlight we’ll see that all the documents are not discoverable as they are image-only and therefore contain no text:
For TermSet to be able to extract metadata from the documents, we need to convert them to searchable PDFs:
After the OCR, if TermSet has been configured properly, it will automatically extract metadata from the newly discoverable documents:
To do this, TermSet goes through the 3 stages described above: Discovery, Analysis and Enrichment. During the Enrichment phase, TermSet creates new term sets and terms based on the information extracted during the Discovery phase. You have full control on which terms to add or exclude using TermSet’s “Taxonomy Mapping” tool:
You can also choose to add the terms in a new term set or an existing one in SharePoint:
Depending on the settings chosen, all the term sets and terms are automatically added to SharePoint’s Taxonomy Term Store.
In the final step of the Enrichment phase, TermSet creates the columns in the document library and populates them with the appropriate terms for each document (metadata tagging). It would not be too unrealistic to say that the above process, if done manually for a larger document set could take months to achieve. Automating it with TermSet can instead take only a fraction of that time. Using TermSet in conjunction with Aquaforest Searchlight can significantly improve the time taken for end-users to find the right information, thus increasing productivity.