Tesseract Python: Extract text from images using Tesseract OCR
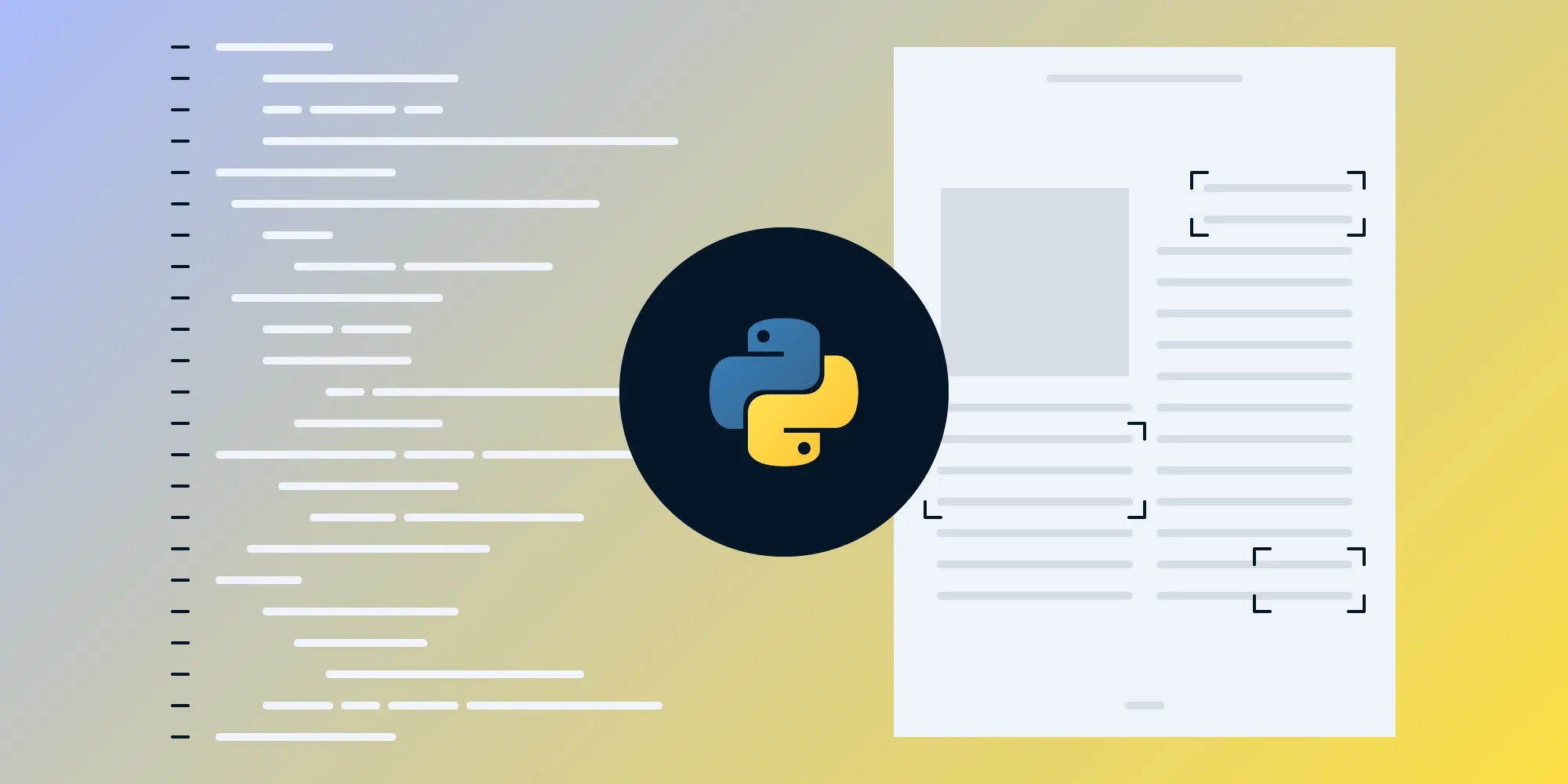
This tutorial covers implementing Tesseract OCR in Python for text extraction from images. Learn installation, basic usage with pytesseract, and accuracy-enhancing techniques like grayscale conversion, resizing, and adaptive thresholding. For complex layouts or poor image quality, Tesseract has limitations. Alternatively, Nutrient OCR API offers advanced features for creating searchable PDFs with better accuracy and layout preservation.
Optical character recognition (OCR)(opens in a new tab) is essential for converting images of text into machine-encoded text, and Python provides powerful tools to streamline this process. In this tutorial, you’ll learn how to utilize Tesseract OCR(opens in a new tab), a robust open source OCR engine, to recognize text from images and scanned documents. You’ll then expand on this by using Nutrient API to enhance your workflow by extracting text and creating searchable PDFs from these documents.
Introduction to optical character recognition
Optical character recognition (OCR) is a technology used to recognize and extract text from images, scanned documents, or other visual media. OCR systems employ a combination of machine learning algorithms and traditional image processing techniques to identify and interpret characters within an image. The extracted text can then be utilized for various purposes, such as data entry, document indexing, and text analysis.
OCR has numerous applications across various industries, including finance, healthcare, education, and government. For instance, in the finance sector, OCR can be used to digitize paper documents, automate data entry, and streamline document management. In healthcare, OCR can help in digitizing patient records and extracting information from medical forms. Educational institutions can use OCR to digitize textbooks and academic papers, making them searchable and easier to manage. Government agencies can leverage OCR to process forms and applications more efficiently.
By converting physical documents into machine-readable text, OCR technology significantly enhances the ability to manage and analyze data, leading to improved efficiency and productivity.
What is Tesseract?
Tesseract OCR(opens in a new tab) is an open source optical character recognition (OCR) engine initially developed by Hewlett-Packard and now maintained by Google.
It’s one of the most widely used and accurate OCR engines available today, applied in diverse fields such as document digitization, image processing, and text extraction. Tesseract OCR uses a combination of machine learning techniques, including neural networks, and traditional image processing methods to accurately recognize text within images. It supports multiple languages and a broad range of fonts, making it versatile for various OCR needs.
Tesseract OCR is licensed under the Apache 2.0 license and can be used as a standalone tool or accessed through APIs for integration into other applications. It’s compatible with several programming languages and frameworks, including Python, Java, and C++. Tesseract also has a large and active community of contributors who continue to enhance its capabilities and provide ongoing support.
Why use Tesseract OCR in Python?
Tesseract OCR supports more than 100 languages and is adept at handling various fonts, sizes, and text styles. Tesseract OCR is commonly used in document analysis, automated text recognition, and image processing, making it a versatile tool for any Python-based OCR project.
Pros and cons of Tesseract OCR
Pros:
- High accuracy — Tesseract is renowned for its precision in text recognition.
- Language support — Supports more than 100 languages, accommodating a wide range of text.
- Versatility — Handles various fonts and text styles effectively.
- Open source — Free to use, with an active community contributing to its development.
- Strong community support — Regular updates, extensive documentation, and a wealth of resources available for developers.
Cons:
- Complex setup — Initial configuration and installation can be challenging for beginners.
- Performance with complex layouts — May struggle with highly complex or non-standard text layouts without additional preprocessing.
- Variable accuracy — Performance can vary depending on image quality and text clarity.
- Limited built-in features — May require additional libraries or tools for advanced functionalities like layout analysis or multi-language support.
Prerequisites
Before beginning, make sure you have the following installed on your system:
- Python 3.x
- Tesseract OCR
- pytesseract(opens in a new tab)
- Pillow (Python Imaging Library)(opens in a new tab)
The pytesseract package is a wrapper for the Tesseract OCR engine that provides a simple interface to recognize text from images. It can also be used as a standalone invocation script to interact directly with the Tesseract OCR engine.
Installing Tesseract OCR
To install Tesseract OCR on your system, follow the instructions for your specific operating system:
- Windows — Download the installer from the official GitHub repository(opens in a new tab) and run it.
- macOS — Use Homebrew by running
brew install tesseract
. - Linux (Debian/Ubuntu) — Run
sudo apt install tesseract-ocr
.
You can find more installation instructions for other operating systems here(opens in a new tab).
Setting up your Python OCR environment
- Create a new Python file in your favorite editor and name it
ocr.py
. - Download the sample image used in this tutorial here and save it in the same directory as the Python file.
- Install the required Python libraries using
pip
:
pip install pytesseract pillow
To verify that Tesseract OCR is properly installed and added to your system’s PATH
, open a command prompt (Windows) or terminal (macOS/Linux) and run the following command:
tesseract --version
You’ll see the version number of Tesseract, along with some additional information. If you encounter issues installing pytesseract, follow these steps to resolve common problems.
How to perform OCR in Python with Tesseract
Now that you’ve installed the pytesseract package, you’ll see how to use it to recognize text from an image.
Import the necessary libraries and load the image you want to extract text from:
import pytesseractfrom PIL import Image
image_path = "path/to/your/image.jpg"image = Image.open(image_path)
Extracting text from the image
To extract text from the image, use the image_to_string()
function from the pytesseract library:
extracted_text = pytesseract.image_to_string(image)print(extracted_text)
The image_to_string()
function takes an image as an input and returns the recognized text as a string.
Run the Python script to see the extracted text from the sample image:
python3 ocr.py
You’ll see the output shown below.
Saving extracted text to a file
If you want to save the extracted text to a file, use Python’s built-in file I/O functions:
with open("output.txt", "w") as output_file: output_file.write(extracted_text)
Advanced Python OCR techniques
In addition to the basic usage, the pytesseract package provides several advanced options for configuring the OCR engine, outlined below.
Configuring the OCR engine
You can configure the OCR engine by passing a configuration string to the image_to_string()
function. The configuration string is a set of key-value pairs separated by a space or a newline character.
For example, the following configuration string sets the language to English and enables the PageSegMode
mode to treat the image as a single block of text:
config = '--psm 6 -l eng'text = pytesseract.image_to_string(image, config=config)
You can also set the path to the Tesseract OCR engine executable using the pytesseract.pytesseract.tesseract_cmd
variable. For example, if the Tesseract OCR engine is installed in a non-standard location, you can set the path to the executable using the following code:
pytesseract.pytesseract.tesseract_cmd = '/path/to/tesseract'
Handling multiple languages
The Tesseract OCR engine supports more than 100 languages. You can recognize text in multiple languages by setting the language option to a comma-separated list of language codes.
For example, the following configuration string sets the language to English and French:
config = '-l eng+fra'text = pytesseract.image_to_string(image, config=config)
Improving OCR accuracy with image preprocessing
To improve the accuracy of OCR, you can preprocess an image before running it through the OCR engine. Preprocessing techniques can help enhance the image quality and make it easier for the OCR engine to recognize text.
Converting images to grayscale
One common preprocessing technique is to convert the image to grayscale. This can help to improve the contrast between the text and the background. Use the grayscale()
method from the ImageOps
module of the Pillow
library to convert the input image to grayscale:
from PIL import Image, ImageOps
# Open an image.image = Image.open("path_to_your_image.jpg")
# Convert image to grayscale.gray_image = ImageOps.grayscale(image)
# Save or display the grayscale image.gray_image.show()gray_image.save("path_to_save_grayscale_image.jpg")
Original image | Grayscale image |
---|---|
![]() | ![]() |
Resizing the image for better accuracy
Another preprocessing technique is to resize the image to a larger size. This can make the text in the image larger and easier for the OCR engine to recognize. Use the resize()
method from the Pillow
library to resize the image:
# Resize the image.scale_factor = 2resized_image = gray_image.resize( (gray_image.width * scale_factor, gray_image.height * scale_factor), resample=Image.LANCZOS)
In the code above, you’re resizing gray_image
to a larger size using a scale factor of 2
. The new size of the image is (width * scale_factor, height * scale_factor)
. This makes use of the Lanczos resampling filter to resize the image, which produces high-quality results.
Applying adaptive thresholding
Adaptive thresholding can help improve OCR accuracy by creating a more binary image with a clear separation between the foreground and background. Use the FIND_EDGES
filter from the ImageFilter
module of the Pillow
library to apply adaptive thresholding to the image:
from PIL import Image, ImageOps, ImageFilter
# Load the image.image = Image.open('image.png')
# Convert the image to grayscale.gray_image = ImageOps.grayscale(image)
# Resize the image to enhance details.scale_factor = 2resized_image = gray_image.resize( (gray_image.width * scale_factor, gray_image.height * scale_factor), resample=Image.LANCZOS)
# Apply edge detection filter (find edges).thresholded_image = resized_image.filter(ImageFilter.FIND_EDGES)
# Save or display the processed image.thresholded_image.show() # This will display the image.# thresholded_image.save('path_to_save_image') # This will save the image.
Original image | Thresholded image |
---|---|
![]() | ![]() |
Finally, you can pass the preprocessed image to the OCR engine to extract the text. Use the image_to_string()
method of the pytesseract
package to extract the text from the preprocessed image:
# Extract text from the preprocessed image.improved_text = pytesseract.image_to_string(thresholded_image)print(improved_text)
Complete OCR script
By using these preprocessing techniques, you can improve the accuracy of OCR and extract text from images more effectively.
Here’s the complete code for the improved OCR script:
from PIL import Image, ImageOps, ImageFilterimport pytesseract
# Define the path to your image.image_path = 'image.png'
# Open the image.image = Image.open(image_path)
# Convert image to grayscale.gray_image = ImageOps.grayscale(image)
# Resize the image to enhance details.scale_factor = 2resized_image = gray_image.resize( (gray_image.width * scale_factor, gray_image.height * scale_factor), resample=Image.LANCZOS)
# Apply adaptive thresholding using the `FIND_EDGES` filter.thresholded_image = resized_image.filter(ImageFilter.FIND_EDGES)
# Extract text from the preprocessed image.improved_text = pytesseract.image_to_string(thresholded_image)
# Print the extracted text.print(improved_text)
# Optional: Save the preprocessed image for review.thresholded_image.save('preprocessed_image.jpg')
Recognizing digits only
Sometimes, you only need to recognize digits from an image. You can set the --psm
option to 6
to treat the image as a single block of text and then use regular expressions to extract digits from the recognized text.
For example, the following code recognizes digits from an image:
import pytesseractfrom PIL import Image, ImageOpsimport re
image_path = "image.png"image = Image.open(image_path)
config = '--psm 6'text = pytesseract.image_to_string(image, config=config)digits = re.findall(r'\d+', text)print(digits)
Here, you import the re
module for working with regular expressions. Then, you use the re.findall()
method to extract all the digits from the OCR output.
Training Tesseract with custom data
Training Tesseract with custom data allows you to fine-tune the OCR engine for specific use cases, fonts, or languages, improving its accuracy in recognizing characters and layouts that may not be well-represented in the default model. Tesseract’s neural network-based recognition engine requires structured training data to learn the distinct patterns of custom characters and fonts.
To train Tesseract with custom data, you’ll need a dataset of images and corresponding text files that contain the desired output text. Tesseract provides tools, such as tesstrain
and text2image
, which assist in generating and labeling training data. Using these tools, you can create a custom language model that Tesseract can use to improve recognition accuracy for specific content.
Though training Tesseract can be time-intensive, it offers significant benefits by tailoring the OCR engine to handle text in unique fonts, symbols, or languages, especially for specialized applications.
Best practices for using Tesseract OCR
To maximize Tesseract’s OCR accuracy and efficiency, consider the following best practices:
Preprocess the input image — Tesseract performs best with clean, high-quality images. Improve the input quality by resizing, converting to grayscale, and applying thresholding or binarization to reduce noise and enhance contrast. This preprocessing can significantly improve recognition rates.
Choose the correct page segmentation mode (PSM) — Tesseract offers several page segmentation modes that analyze different image layouts. Selecting the appropriate mode can help the engine better interpret text arrangements, such as dense paragraphs, sparse words, or complex layouts.
Specify the correct language — Tesseract supports a wide range of languages. Specifying the correct language using the
-l
flag (e.g.-l eng
for English) improves the OCR accuracy by narrowing down language-specific characters and patterns.Use a custom language model if needed — For text in rare languages, custom symbols, or unique fonts, creating a custom language model can significantly boost accuracy. This model allows Tesseract to better interpret characters specific to your application.
Test and evaluate the results — Regular testing and evaluation help ensure the OCR engine performs as expected. You can use Tesseract’s evaluation tools, or external ones, to assess accuracy and make adjustments as needed.
By following these best practices, you can optimize Tesseract OCR for your project, improving both accuracy and efficiency in recognizing text.
Troubleshooting import errors with pytesseract
Issues importing pytesseract can arise from installation problems, version conflicts, or environment misconfigurations. This guide will help you resolve common issues.
Common causes of pytesseract import errors
- Incorrect Installation
- Ensure that pytesseract is installed in the correct Python environment.
- Verify installation by running:
pip show pytesseract
If it’s not installed, install it using:
pip install pytesseract
- Multiple Python versions
If you have multiple versions of Python installed, ensure that pytesseract
is installed in the environment corresponding to the Python version you’re using.
- Check your Python version with:
python3 --version
- Use the correct pip version:
python3 -m pip install pytesseract
- Environment issues
- If you’re using virtual environments, activate the correct environment before installing or running your script.
- Check if the environment is activated:
source your_env_name/bin/activate
Install pytesseract
within the activated environment.
- System path issues
- Ensure that Python and pip paths are correctly set in your system environment variables.
- Check your current Python path:
which python3
Additional tips
- Reinstall
pytesseract
— If problems persist, try uninstalling and reinstallingpytesseract
:
pip uninstall pytesseractpip install pytesseract
- Check Tesseract installation — Ensure Tesseract is correctly installed on your system. You can verify this by running:
tesseract --version
- Upgrade pip — Sometimes, simply upgrading pip can resolve issues:
python3 -m pip install --upgrade pip
Install packages on managed environments — If you’re encountering issues installing packages due to an externally managed environment (like macOS with Homebrew), follow the steps outlined below.
- Use a virtual environment:
Terminal window python3 -m venv myenvsource myenv/bin/activatepip install pytesseract- Use
pipx
(opens in a new tab) for isolated environments:
Terminal window brew install pipxpipx install pytesseract- Override the restriction (not recommended):
Terminal window python3 -m pip install pytesseract --break-system-packages
Check PEP 668(opens in a new tab) for more details on this behavior.
By following these steps, you can effectively troubleshoot and resolve most issues related to importing pytesseract in Python.
Limitations of Tesseract
While Tesseract OCR is a powerful and widely used OCR engine, it has some limitations and disadvantages that are worth considering. Here are a few of them:
- Accuracy can vary — While Tesseract OCR is generally accurate, the accuracy can vary depending on the quality of the input image, the language being recognized, and other factors. In some cases, the OCR output may contain errors or miss some text altogether.
- Training is required for non-standard fonts — Tesseract OCR works well with standard fonts, but it may have difficulty recognizing non-standard fonts or handwriting. To improve recognition of these types of fonts, training data may need to be created and added to Tesseract’s data set.
- Limited support for complex layouts — Tesseract OCR works best with images that contain simple layouts and clear text. If the image contains complex layouts, graphics, or tables, Tesseract may not be able to recognize the text accurately.
- Limited support for languages — While Tesseract OCR supports many languages, it may not support all languages and scripts. If you need to recognize text in a language that isn’t supported by Tesseract, you may need to find an alternative OCR engine.
- No built-in image preprocessing — While Tesseract OCR can recognize text from images, it doesn’t have built-in image preprocessing capabilities. Preprocessing tasks like resizing, skew correction, and noise removal may need to be done separately before passing the image to Tesseract.
Nutrient API for OCR
Nutrient’s OCR API allows you to process scanned documents and images to extract text and create searchable PDFs. This API is designed to be easy to integrate into existing workflows, and the well-documented APIs and code samples make it simple to get started.
Nutrient’s OCR API provides the following benefits:
- Generate interactive PDFs via a single API call for scanned documents and images.
- SOC 2 compliance ensures workflows can be built without any security concerns. No document data is stored, and API endpoints are served through encrypted connections.
- Access to more than 30 tools allows processing one document in multiple ways by combining API actions such as conversion, OCR, rotation, and watermarking.
- Simple and transparent pricing, where you only pay for the number of documents processed, regardless of file size, datasets being merged, or different API actions being called.
Requirements
To get started, you’ll need:
To access your Nutrient API key, sign up for a free account(opens in a new tab). Once you’ve signed up, you can find your API key in the Dashboard > API Keys section(opens in a new tab).
Python is a programming language, and pip is a package manager for Python, which you’ll use to install the requests
library. Requests(opens in a new tab) is an HTTP library that makes it easy to make HTTP requests.
Install the requests
library with the following command:
python3 -m pip install requests
Using the OCR API
- Import the
requests
andjson
modules:
import requestsimport json
- Define OCR processing instructions in a dictionary:
instructions = { 'parts': [{'file': 'scanned'}], 'actions': [{'type': 'ocr', 'language': 'english'}]}
In this example, the instructions specify a single part ("file": "scanned"
) and a single action ("type": "ocr", "language": "english"
).
- Send a
POST
request to the Nutrient API endpoint to process the scanned document:
response = requests.request( 'POST', 'https://api.pspdfkit.com/build', headers={ 'Authorization': 'Bearer <YOUR API KEY HERE>' }, files={'scanned': open('image.png', 'rb')}, data={'instructions': json.dumps(instructions)}, stream=True)
Replace <YOUR API KEY HERE>
with your API key.
Here, you make a request to the Nutrient API, passing in the authorization token and the scanned document as a binary file. You also pass the OCR processing instructions as serialized JSON data.
You can use the demo image here to test the OCR API.
- If the response is successful (status code
200
), create a new searchable PDF file from the OCR-processed document:
if response.ok: with open('result.pdf', 'wb') as fd: for chunk in response.iter_content(chunk_size=8096): fd.write(chunk)else: print(response.text) exit()
Advanced OCR: Merging scanned pages into a searchable PDF with Nutrient API
In addition to running OCR on a single scanned page, you may have a collection of scanned pages you want to merge into a single searchable PDF. Fortunately, you can accomplish this by submitting multiple images, with one for each page, to Nutrient API.
To test this feature, add more files to the same folder your code is in, and modify the existing code accordingly. You can duplicate and rename the existing file or use other images containing text:
import requestsimport json
instructions = { 'parts': [ { 'file': 'page1.jpg' }, { 'file': 'page2.jpg' }, { 'file': 'page3.jpg' }, { 'file': 'page4.jpg' } ], 'actions': [ { 'type': 'ocr', 'language': 'english' } ]}
response = requests.request( 'POST', 'https://api.pspdfkit.com/build', headers = { 'Authorization': 'Bearer <YOUR API KEY HERE>' }, files = { 'page1.jpg': open('page1.jpg', 'rb'), 'page2.jpg': open('page2.jpg', 'rb'), 'page3.jpg': open('page3.jpg', 'rb'), 'page4.jpg': open('page4.jpg', 'rb') }, data = { 'instructions': json.dumps(instructions) }, stream = True)
if response.ok: with open('result.pdf', 'wb') as fd: for chunk in response.iter_content(chunk_size=8096): fd.write(chunk)else: print(response.text) exit()
Replace <YOUR API KEY HERE>
with your API key.
The OCR API will merge all of the input files into a single PDF before running OCR on it. The resulting PDF is then returned as the response content.
Conclusion
In this post, you explored how to leverage Tesseract OCR in Python for extracting text from images and scanned documents. It covered the basics of setting up Tesseract, using the pytesseract library for text extraction, and enhancing accuracy with image preprocessing techniques such as grayscale conversion, resizing, and adaptive thresholding. Additionally, you learned about integrating Nutrient’s OCR API to process documents and create searchable PDFs efficiently.
By applying these techniques and tools, you can automate and optimize your text extraction workflows, regardless of whether you’re dealing with simple documents or complex layouts. Combining the power of Tesseract OCR and Nutrient’s advanced capabilities opens up new possibilities for handling and analyzing text data in your projects.
Feel free to experiment with different preprocessing methods and configurations to tailor the OCR process to your specific needs and achieve the best results for your text extraction tasks.
FAQ
What is Tesseract OCR?
Tesseract OCR is an open source engine for recognizing text from images and scanned documents. Developed by Hewlett-Packard and now sponsored by Google, it supports more than 100 languages and various text styles.
How do I install Tesseract OCR in Python?
To install Tesseract OCR, download the installer from GitHub for Windows(opens in a new tab), use brew install tesseract
on macOS, or run sudo apt install tesseract-ocr
on Debian/Ubuntu.
How can I improve OCR accuracy?
You can improve OCR accuracy by converting an image to grayscale, resizing it to make the text larger, and applying adaptive thresholding to enhance text contrast.
Can Tesseract OCR handle multiple languages?
Yes, Tesseract supports multiple languages. Use a plus sign (+
) in the configuration string, like -l eng+fra
for English and French.
What are the limitations of Tesseract OCR?
Tesseract’s limitations include varying accuracy based on image quality, difficulty with non-standard fonts, and limited support for complex layouts and languages. It also lacks built-in image preprocessing.
How does Nutrient’s OCR API work?
Nutrient’s OCR API extracts text from scanned documents and creates searchable PDFs. It offers features like interactive PDFs, SOC 2 compliance, and transparent pricing.
How do I use Nutrient’s OCR API?
Install the requests
library with python3 -m pip install requests
. Define OCR instructions in a dictionary, and then send a POST
request to https://api.pspdfkit.com/build
with your API key and document. Save the result if the response is successful.
Can I merge multiple scanned pages into one searchable PDF using Nutrient?
Yes, you can merge multiple images into a single searchable PDF. Adjust the file handling and instructions in your API request to include all pages.